According to Forbes (via Gartner), 85% of AI projects in strategic sectors fail due to transparency issues, and understanding how artificial intelligence makes decisions is a significant challenge. This lack of clarity is particularly concerning in the healthcare, finance, and law enforcement industries, where AI decisions can have profound consequences.
The importance of transparency in AI cannot be overstated; it is a technical necessity and a foundation for building trust. Users’ confidence in these technologies increases substantially when they understand how AI systems work.
The solution to this challenge lies in promoting visibility when designing and deploying AI systems. This includes using frameworks that ensure explainability and accountability throughout the AI lifecycle. By improving data governance and providing clear explanations for AI decisions, organizations can build trust and unlock the full potential of these technologies. Transparent AI systems are more reliable and better equipped to address ethical and societal challenges, preparing for responsible innovation.
Let’s understand the reasoning model
AI reasoning models are specialized systems that simulate logical processes, often relying on explicit knowledge representation and inference mechanisms. These models are crucial for handling complex tasks requiring calculation, thought, and analysis.
Unlike traditional chat models, reasoning models engage in “inference-time computing,” where they pause to evaluate scenarios methodically. This intentional approach allows reasoning models to address complex problems, such as diagnosing medical conditions, planning tasks, or analyzing legal cases.
Large Language Models (LLMs) have transformed the AI environment by enabling machines to process and generate human-like text. However, traditional LLMs often operate as “black boxes,” providing results without revealing the underlying logic. Reasoning models incorporate techniques like Chain of Thought (CoT) prompting that enhances the symbolic reasoning capabilities of LLMs by encouraging them to break down problems into manageable steps, helping them follow through with the rules or logical process needed to reach correct conclusions. This phased approach improves transparency and trustworthiness by allowing AI systems to articulate their internal thought processes.
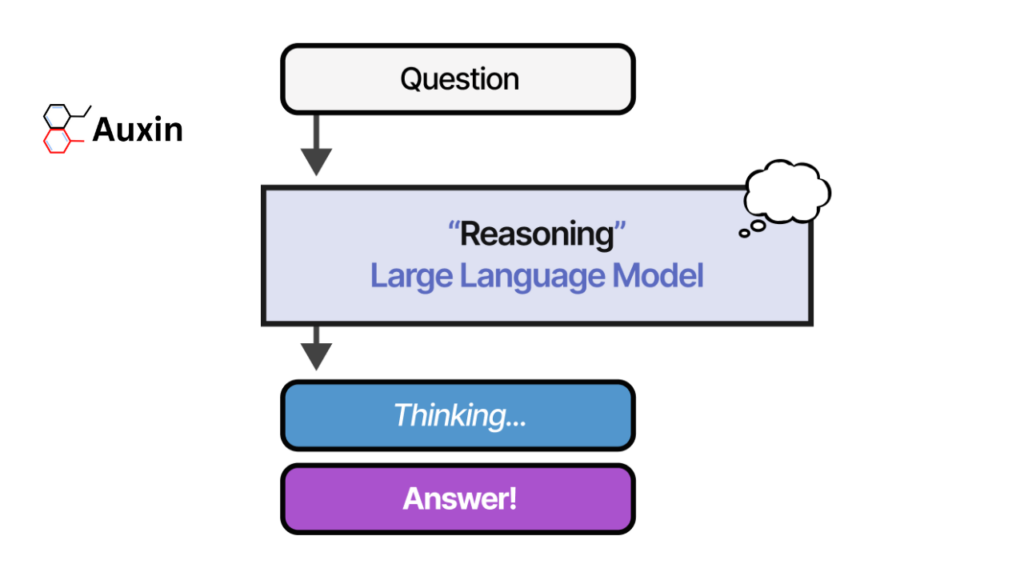
7 Types of Reasoning
Here are seven types of reasoning and examples of situations when they’re best used:
- Deductive Reasoning
It starts with a general assumption and uses logic to prove or disprove it. The results are logically specific. For example, a company identifies young parents as its biggest audience and invests in social media targeting them.
- Inductive Reasoning
Observes specific cases to form general rules. Results are not always exact but are helpful for predictions. For example, a teacher notices better student focus after adding a short break and making it a routine.
- Analogical Reasoning
Compare the similarities between the two things to conclude. Relating new concepts to familiar ones helps in understanding them. For example, a business uses a supermarket analogy to meet all customer needs.
- Abductive Reasoning
It makes the best guess based on limited observations and is often used in uncertain situations. For example, a salesperson guesses a client’s issue based on minimal information and prepares potential solutions.
- Cause-and-Effect Reasoning
Link actions to outcomes, predicting results based on past experiences or trends. For example, a marketing agency shows how increased ad spending led to higher sales, justifying further budget increases.
- Critical Thinking
Analyzes and evaluates information deeply to make informed decisions, especially in complex or uncertain scenarios. For example, a manager plans alternatives when a key supplier announces a strike.
- Syllogistic Reasoning
It uses logical connections between premises to conclude, though accuracy depends on the truth of the premises. Example: “All birds fly; penguins are birds; therefore, penguins fly” (an incorrect conclusion due to a false belief).
These reasoning types improve decision-making, problem-solving, and critical thinking skills.
Chain of Thought and Slow Thinking
Chain of Thought (CoT) reasoning is an AI method that breaks complex problems into step-by-step sequences, improving transparency and accuracy. This approach allows AI systems to clear their thought processes, enhancing trust and interpretability. For example, CoT can explain decisions like loan approvals by detailing each step. Models like ReACT use CoT traces to generate insights iteratively.
CoT bridges fast heuristics (System 1 thinking) with slow, deliberate analysis (System 2), mirroring human cognition. Slow thinking enables AI to tackle complex tasks by breaking them into manageable steps, ensuring precise results. Auxin Security improves CoT frameworks with strong security measures to protect sensitive data.
Fast and Slow AI Thinking
LLMs can be understood through Daniel Kahneman’s work on System 1 and System 2 thinking. System 1 thinking is fast, intuitive, and reactive, excelling at quick responses and conversational tasks. In contrast, System 2 thinking is slow, deliberate, and analytical, ideal for solving complex math problems or analyzing research papers. Reasoning models are designed to engage in System 2 thinking, taking longer to respond because they methodically break down problems into manageable steps.
For example, fast LLMs (System 1) show expected outcome solutions but do not reveal the actual reasoning trace in their next-token prediction. Slow LLMs (System 2) use consequential thinking by breaking down problems into concrete plans and executing them until they reach a highly likely answer.
A reasoning trace is a step-by-step process language models use to articulate their internal thought patterns while solving a task. Auxin Security provides secure infrastructure for LLMs to operate efficiently while protecting reasoning processes.
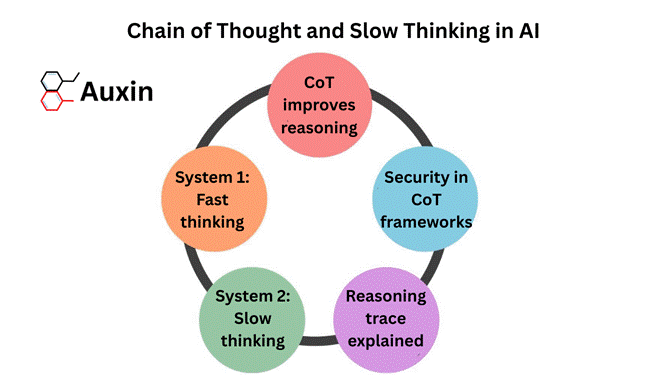
What’s Next for AI Reasoning
Balancing fast and slow thinking is crucial for AI evolution. Fast models are intuitive but less transparent, while slow models offer detailed reasoning for high-stakes decisions. Frameworks like CoT integrate these approaches and enable AI to handle diverse workloads effectively.
Advancements like OpenAI’s O3 Mini demonstrate the potential of CoT in industries such as healthcare and finance by combining efficient retrieval and structured problem-solving. Transparent AI systems equipped with CoT reasoning will address ethical challenges, build trust, and drive innovation across sectors.
This technique ensures AI systems are efficient and reliable in solving complex problems while maintaining transparency. Auxin Security supports these advancements by integrating security solutions adapted to industry-specific needs.
Let’s Sum it up
Transparency and explainability are crucial for trustworthy AI systems, especially in strategic industries like healthcare and finance. Chain-of-thought reasoning improves interpretability by breaking down problems into manageable steps, while frameworks like Explainable AI ensure accountability in decision-making processes.
Auxin Security plays a significant role by providing secure environments, strong governance, and specific solutions that empower organizations to adopt transparent, reliable, and innovative AI technologies across diverse sectors.