Trust in the Bytes: Upholding Ethical AI/ML with Data Protection
Auxin agrees with the Boston Consulting Group that AI is the future, but C-Suite needs to a business case at scale. Artificial Intelligence (AI) and Machine Learning (ML) are revolutionizing various industries and transforming businesses’ operations.
With the advent of AI/ML, companies are using large amounts of data to improve customer experiences, increase operational efficiencies, and make better decisions. However, the more data we collect, the greater the risk of data breaches and privacy violations.
Data protection is an essential aspect of AI/ML development that must be considered. The sensitivity of the data being used, the scale of processing, and the complexity of algorithms make data protection in AI/ML particularly challenging. The consequences of data breaches in AI/ML can be severe, including reputational damage, legal liability, and financial losses.
Therefore, it is critical to ensure that AI/ML applications are designed with robust data protection mechanisms that guarantee the privacy and security of data throughout its lifecycle.
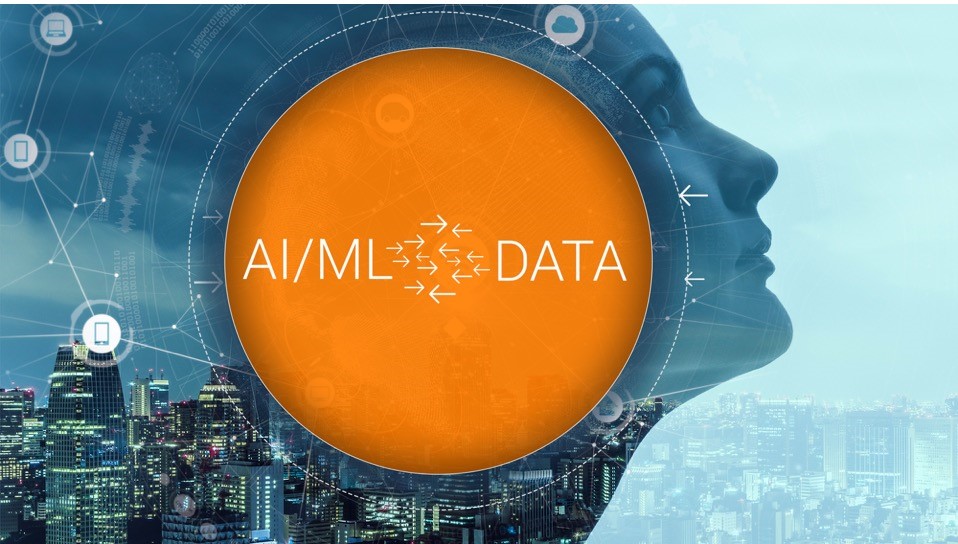
Risks of Data Breaches in AI/ML Applications
Data breaches are one of the most significant risks associated with advanced applications. The massive amount of data used in these applications and their complex algorithms makes them particularly vulnerable to cyber threats. Here are some potential risks of data breaches in AI/ML applications:
- Unauthorized Access: Hackers and cybercriminals can gain access to these systems and steal sensitive data, such as privately identifiable information (PII), financial information, and intellectual property.
- Malicious Attacks: Malicious actors can launch attacks against systems to manipulate the data, inject malicious code, or compromise the algorithms.
- Bias and Discrimination: These systems trained on partial data can perpetuate and amplify discrimination, leading to unfair and unethical outcomes.
- Regulatory Non-Compliance: Data breaches can result in legal and regulatory consequences, such as fines and reputational damage, for organizations failing to comply with data privacy and security regulations.
- Loss of Intellectual Property: These systems may process and store valuable intellectual property, such as trade secrets, patents, and proprietary data. A data breach can result in the loss or theft of this intellectual property, causing significant damage to an organization’s competitiveness and innovation.
To mitigate these risks, organizations must take proactive measures to secure their AI/ML systems, including robust data protection mechanisms, regular security assessments, and compliance with applicable regulations.
Best Practices for Securing Data in AI/ML
Securing data in AI/ML is essential to protect sensitive information from data breaches and maintain data privacy compliance. Here are some best practices for securing data in AI/ML:
- Data Encryption: Data encryption is encoding sensitive data to prevent unauthorized access. End-to-end encryption in AI/ML systems can protect sensitive data from cyber-attacks.
- Access Control: Access control limits access to sensitive data based on roles, responsibilities, and privileges. Implementing strict access control measures can prevent unauthorized access to sensitive data.
- Data Anonymization: Data anonymization is a process of removing personally identifiable information from datasets, thereby making it impossible to identify individuals. This practice helps protect the privacy of individuals in datasets used in AI/ML.
- Regular Data Audits: Regular data audits can help organizations determine potential vulnerabilities and weaknesses in their data protection mechanisms. It can also help ensure compliance with data privacy regulations.
- Data Backup and Recovery: Implementing regular data backup and recovery procedures can help ensure that data is recoverable in case of a data breach or loss.
- Robust Security Framework: Implementing a robust security framework can help organizations identify potential security risks, such as cyber-attacks and data breaches, and take proactive measures to prevent them.
- Secure Data Transmission: Data transmission over networks is a vulnerable point in the data lifecycle. Therefore, organizations must secure data transmission using encryption and other security measures.
By implementing these best practices, organizations can ensure that their AI/ML systems are secure and compliant and safeguard sensitive data throughout their lifecycle.
Conclusion
Data protection is critical for organizations developing AI/ML applications. These systems process and store large amounts of sensitive data, making them particularly vulnerable to cyber-attacks and data breaches. Organizations must prioritize data protection throughout the AI/ML development lifecycle to mitigate these risks and ensure compliance with data privacy regulations. For more knowledge read our blogs on our website Auxin.io.