Recently Gartner published interesting research titled “ Hype Cycle for Artificial Intelligence, 2021”; predictive AI is a well-established technology currently in the “plateau of productivity” phase, indicating its widespread adoption and practical application in various industries. The most recent Gartner Hype Cycle 2021 estimate that analytics is a well-established technology currently in the “plateau of productivity” phase, indicating its widespread adoption and practical application in various industries.
On the other hand, generative AI is still in the “innovation trigger” phase, meaning it is still in the formative years of development and has not yet reached widespread adoption.
Gartner predicts that generative AI will become increasingly important in the coming years, particularly in the entertainment, design, and healthcare industries. In contrast, predictive AI will continue to be used in finance, marketing, and supply chain management, where it has already demonstrated significant value.
Auxin Security agrees with Gartner, and we see both predictive and generative AI as essential tools in the toolkit, each with strengths and use cases. In this article, Auxin explains the difference between predictive and generative AI to help organizations understand the technology use cases.
The type of AI is a fundamental question and should be the initial focus of all organizations based on their business use-case, requirements, and goals. Organizations will need to understand the capabilities and limitations of each type of AI and choose the right approach based on their specific needs and goals.
Predictive AI
Predictive artificial intelligence (AI) is a type of machine learning that uses historical data and statistical algorithms to identify patterns and predict future events or behaviors. It has a wide range of use cases and has proven to be a priceless tool for businesses and organizations across many industries.
Generative AI
Generative artificial intelligence (AI) is a type of machine learning that involves creating entirely new and unique content based on input data. Unlike predictive AI, which uses historical data to predict future events, generative AI can create new content, such as images, music, or text, based on patterns and trends identified in the input data.
Predictive AI | Generative AI | |
Use-Cases | One of the most shared use cases is in finance, where it is used for fraud detection, risk management, and investment forecasting. In marketing, predictive AI is used to personalize advertisements and recommend products to customers based on browsing history and preferences. In healthcare, it is used for patient risk assessment, disease diagnosis, and treatment recommendations. Predictive AI is also used in supply chain management to forecast demand, optimize inventory levels, and reduce costs. | Generative AI has many use cases, particularly in the entertainment, design, and healthcare industries. In the entertainment industry, it is used for video game design, virtual reality experiences, and special effects in movies and television. In practice, generative AI creates new products and prototypes and optimizes existing structures. In healthcare, it is used for drug discovery and personalized medicine and to generate synthetic medical images for training. |
Pros | The most significant advantage of predictive AI is that it can help businesses make better decisions based on data-driven insights. By analyzing large amounts of historical data, predictive AI can identify patterns and trends that are not immediately apparent to humans. This can help businesses forecast demand, identify potential risks, and make better investment decisions. Predictive AI can also help enterprises to automate specific processes, such as fraud detection, which can save time and money. | The most significant advantage of generative AI is its ability to create entirely new content based on input data. This can be incredibly useful for industries such as entertainment and design, where creativity and innovation are highly valued. Generative AI can also automate specific processes, such as music composition, which can save time and money. Additionally, generative AI can be used for research and development, allowing scientists and researchers to generate new ideas and hypotheses based on large datasets. |
Cons | One of the main challenges of predictive AI is the potential for bias. Predictive AI algorithms are only as good as the training data and if the data is biased, the algorithm will also be personal. This can lead to inaccurate predictions and unfair outcomes, particularly in industries such as finance and healthcare, where decisions can have serious consequences. Additionally, predictive AI can be expensive and requires significant data to train the algorithm. | Like predictive AI, generative AI algorithms are only as good as the data they found and trained on. This can cause imprecise or discriminatory outcomes, particularly in industries such as healthcare, where decisions can have serious consequences. Additionally, generative AI can be expensive and requires significant computing power to generate high-quality content. |
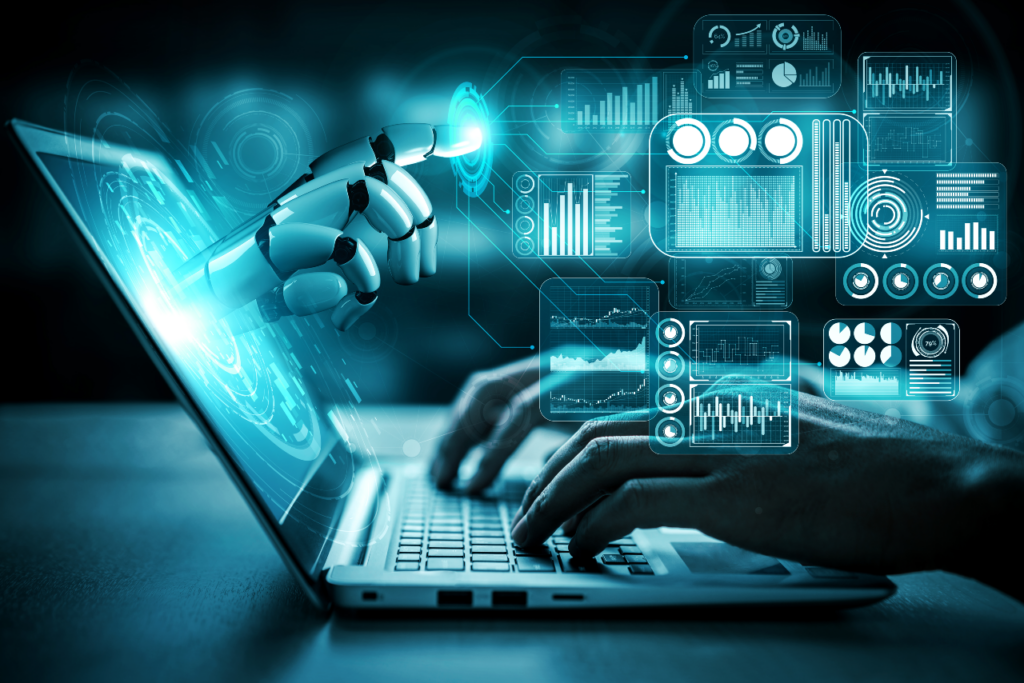
Security Concerns
Predictive and generative AI comes with security concerns, although they differ in their risks. Predictive AI can be vulnerable to attacks such as data poisoning and adversarial attacks, where malicious actors manipulate the input data to affect the predictions made by the algorithm. In addition, predictive AI algorithms can be used for nefarious purposes such as automated fraud or cyber-attacks.
On the other hand, generative AI can create convincing fake content, such as deep fakes, which can be used for wrong purposes, such as spreading disinformation or impersonation. It is essential for organizations using either type of AI to implement robust security measures, such as data encryption and access controls, to minimize the risk of breaches and attacks. Additionally, organizations must stay current with emerging threats and continually assess and adapt their security protocols in response to new risks.
Future
Predictive and generative AI’s future is bright, with many exciting developments and innovations. Predictive AI will likely become even more accurate and efficient with improved algorithms and access to more extensive and diverse datasets. This will lead to new applications and use cases, such as improved personalized medicine and more effective fraud detection.
Meanwhile, generative AI is expected to continue pushing the boundaries of creativity and innovation, with breakthroughs in music composition, product design, and drug discovery. However, as these technologies continue to advance, it will be essential to address their use’s ethical and societal implications, such as bias, transparency, and the impact on jobs and employment.
Overall, the future of predictive and generative AI is exciting and full of potential but must be navigated carefully and responsibly to realize its full benefits.
Conclusion
In Auxin Security‘s opinion, predictive and generative AI represent fascinating and promising developments in artificial intelligence. While predictive AI has already made significant strides in healthcare and fraud detection, much room remains for improvement and innovation. On the other hand, generative AI has the potential to revolutionize creative industries. Its ability to generate new content based on patterns and trends in input data is genuinely remarkable.
As with any emerging technology, some risks and challenges must be addressed, particularly bias, transparency, and security. We are excited to see how these technologies continue to develop and evolve. I believe that responsible and ethical use can bring about significant benefits for individuals, businesses, and society.